Autonomous Driving: NVIDIA's New Approach
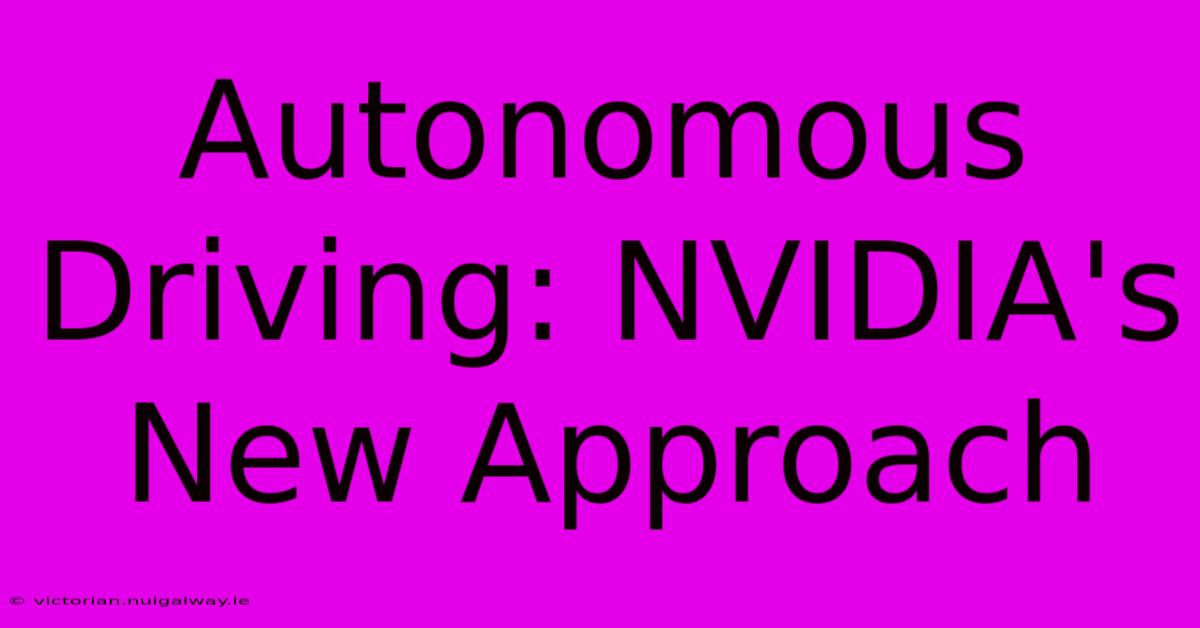
Discover more detailed and exciting information on our website. Click the link below to start your adventure: Visit Best Website. Don't miss out!
Table of Contents
Autonomous Driving: NVIDIA's New Approach
Okay, buckle up, buttercup, because we're diving headfirst into the wild world of self-driving cars – and specifically, NVIDIA's seriously ambitious take on it. Forget everything you think you know about robotic chauffeurs; NVIDIA is shaking things up. This isn't just incremental improvement; it's a paradigm shift.
The Old Guard: Why It Wasn't Enough
For years, the autonomous driving race looked like a Formula 1 competition – everyone meticulously tweaking their existing engines. Companies focused on painstakingly mapping every street, creating intricate rulebooks for their vehicles, and relying on massive data sets. The problem? This approach is brittle, expensive, and incredibly slow to scale. Imagine trying to build a map of the entire world, street by street, and then programming a car to understand every nuance of human behavior on every possible scenario. It's a monumental task. And frankly, a bit insane.
The Limitations of Traditional Mapping
Think about it: a single fallen tree branch, a sudden detour, a rogue shopping cart – these seemingly insignificant events can completely derail a system relying solely on precise maps and pre-programmed rules. The complexity is overwhelming. We're talking about solving a problem that's, frankly, more complicated than predicting the weather.
The Data Deluge and Its Drawbacks
Then there's the data issue. These systems require mountains of data – terabytes upon terabytes – to train their algorithms. Collecting, processing, and storing this data is incredibly expensive and energy-intensive. It's like trying to build a house using only grains of sand – painstaking, inefficient, and prone to collapse.
NVIDIA's Revolution: A Different Kind of Map
NVIDIA’s approach is radically different. They're not just building better maps; they're building a completely new kind of map. Instead of meticulously detailed road layouts, they're focusing on creating a semantic map – a map that understands the meaning of the world, not just its appearance.
Semantic Mapping: Understanding the World, Not Just Seeing It
Imagine the difference between a photograph and a painting. A photograph simply records what's there; a painting interprets it. NVIDIA's semantic maps do the same. They don't just show roads and buildings; they understand the function of those things. They understand that a stop sign means stop, a pedestrian crossing means yield, and a construction zone means proceed with caution.
The Power of AI and Deep Learning
This semantic understanding is made possible through the power of deep learning and artificial intelligence. NVIDIA’s DRIVE software platform leverages advanced neural networks to process sensor data from cameras, lidar, radar, and ultrasonic sensors – creating a rich, contextual understanding of the driving environment.
Beyond the Map: The Importance of Simulation
NVIDIA isn’t stopping at semantic maps. They’re also investing heavily in simulation. Think of it as a virtual proving ground for autonomous vehicles. By simulating countless driving scenarios – from sunny highways to blizzard-covered streets – they can train their AI systems far more efficiently and safely than ever before.
The Benefits of Simulated Driving
This approach significantly reduces the need for real-world testing, saving time, money, and reducing the risk of accidents during development. It's a bit like training a pilot in a flight simulator before they ever set foot in a real plane.
The DRIVE Constellation: A Game Changer
The key to this new approach is NVIDIA's DRIVE Constellation, a powerful simulation platform capable of generating incredibly realistic and complex driving scenarios. Think of it as the ultimate driving simulator, but on a scale never before seen. It allows engineers to test and refine their algorithms in a safe and controlled environment, accelerating the development process significantly.
Scaling Up: From Simulation to Reality
The beauty of this approach lies in its scalability. Once the AI is trained in simulation, it can be deployed to real-world vehicles with minimal further adjustments. This significantly reduces the time and cost associated with bringing autonomous driving technology to market.
The Future of Autonomous Driving: A Collaborative Approach
NVIDIA's strategy isn't just about building better self-driving cars; it's about building a more collaborative ecosystem. They’re working with a vast network of automakers, technology companies, and researchers to accelerate the development and adoption of autonomous driving technology. This is a marathon, not a sprint, and they know it takes a village.
Addressing Ethical Concerns
Of course, the development of autonomous driving technology raises significant ethical questions. How will these vehicles make decisions in complex or ambiguous situations? How will liability be determined in the event of an accident? These are vital issues that need to be addressed, and NVIDIA is actively participating in the global conversation surrounding these important ethical considerations.
Conclusion: A New Era of Mobility
NVIDIA’s approach to autonomous driving marks a paradigm shift. By embracing semantic mapping, advanced simulation, and a collaborative approach, they’re paving the way for a safer, more efficient, and more accessible future of mobility. It's a journey, not a destination, and the ride promises to be extraordinary.
FAQs
-
How does NVIDIA's semantic mapping differ from traditional mapping techniques? Traditional mapping focuses on precise geometric representations of the environment, whereas NVIDIA's semantic mapping emphasizes understanding the meaning and function of objects within the environment. This allows for greater adaptability and robustness in unpredictable conditions.
-
What is the role of simulation in NVIDIA's autonomous driving strategy? Simulation allows NVIDIA to train and test their AI algorithms in a safe and controlled environment, reducing the need for extensive real-world testing and significantly accelerating the development process.
-
How does NVIDIA's approach address the scalability challenges of autonomous driving? By training AI models extensively in simulation, NVIDIA reduces the reliance on massive real-world datasets, making the technology more scalable and cost-effective to deploy.
-
What ethical considerations are involved in the development of autonomous driving technology, and how is NVIDIA addressing them? Ethical considerations include decision-making in complex scenarios, liability in the event of accidents, and data privacy. NVIDIA actively collaborates with researchers, policymakers, and industry partners to address these concerns.
-
How does NVIDIA's collaborative approach benefit the broader autonomous driving ecosystem? NVIDIA's open platform and partnerships with various stakeholders foster innovation, accelerate technology development, and encourage standardization, leading to faster adoption and wider accessibility of autonomous driving technology.
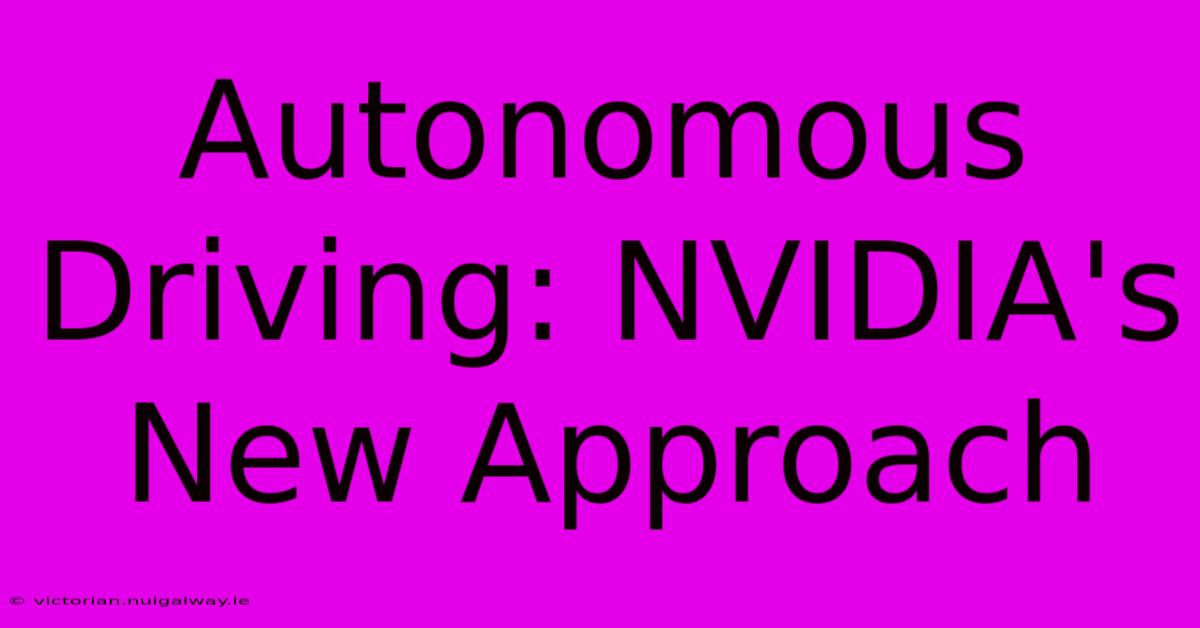
Thank you for visiting our website wich cover about Autonomous Driving: NVIDIA's New Approach. We hope the information provided has been useful to you. Feel free to contact us if you have any questions or need further assistance. See you next time and dont miss to bookmark.
Also read the following articles
Article Title | Date |
---|---|
Winter Graduates Your Microsoft 365 Plan | Jan 07, 2025 |
Nfl Playoffs Rams Vs Vikings | Jan 07, 2025 |
Jets Coaching Job Rex Ryans Plan For Aaron Rodgers | Jan 07, 2025 |
Aaron Rodgers And The Jets A Rex Ryan Perspective | Jan 07, 2025 |
Ancelottis Lineup Decision Real Madrid Vs Deportivo | Jan 07, 2025 |
Lopeteguis West Ham Tenure Uncertain | Jan 07, 2025 |
London Church Pew Removal Marks Change | Jan 07, 2025 |
Ge Force Rtx 50 Boosting Creative Work | Jan 07, 2025 |
Uk Schools Transport Hit By Heavy Snow | Jan 07, 2025 |
Inauguration Day Trumps Presidential Start | Jan 07, 2025 |