Investigation Update: Solebury Model
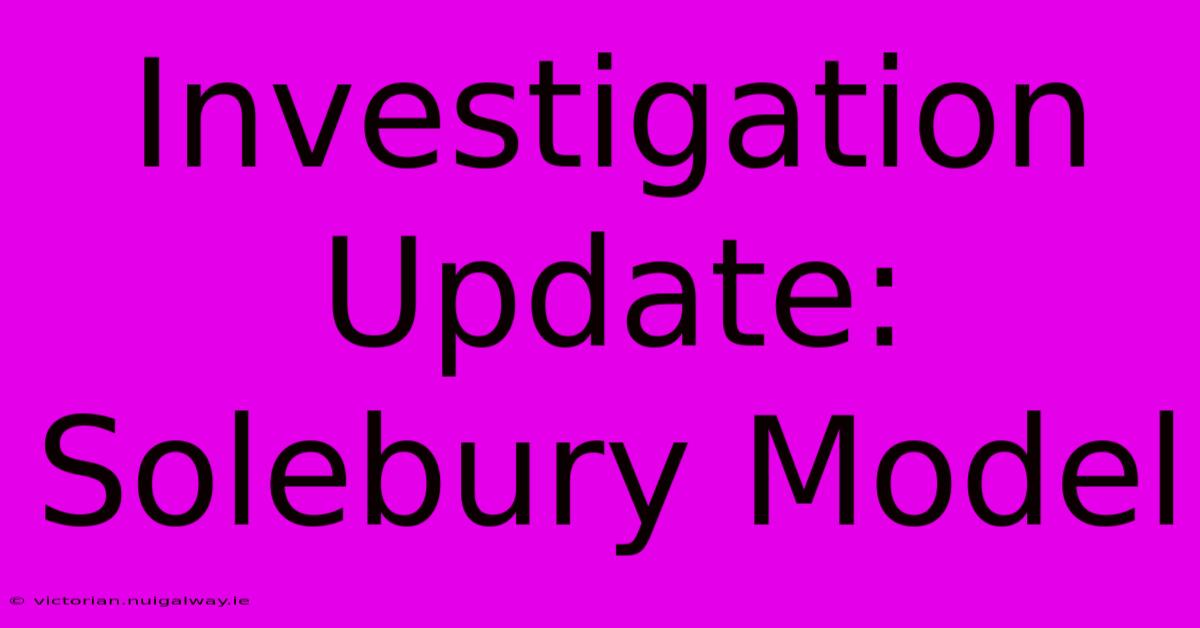
Discover more detailed and exciting information on our website. Click the link below to start your adventure: Visit Best Website. Don't miss out!
Table of Contents
Investigation Update: Solebury Model – Unraveling the Mystery
So, you've heard whispers, maybe seen some frantic tweets, about the Solebury Model. It's the hot new thing, or maybe the not-so-hot new thing, depending on who you ask. Let's dive in, shall we? This isn't your grandma's econometric model; this is something…else. And the investigation into its effectiveness is far from over.
The Hype and the Hope: What is the Solebury Model Supposed to Do?
The Solebury Model, at its core, promises to predict market fluctuations with unnerving accuracy. It’s marketed as a revolutionary approach, leveraging advanced algorithms and a frankly bewildering array of data points – everything from satellite imagery of parking lots (apparently, empty spaces correlate with consumer confidence…who knew?) to social media sentiment analysis about obscure reality TV shows.
A Cocktail of Data: More Than Meets the Eye
The model's creators boast an unprecedented level of granularity. They're not just looking at traditional economic indicators; they're trying to capture the zeitgeist, the collective mood, the subtle shifts in human behavior that might foreshadow market swings. Think of it as a super-powered, data-driven crystal ball.
The Algorithm's Black Box: Understanding the Unknowable
But herein lies the problem. The specifics of the Solebury Model’s algorithm are, shall we say, guarded. It's a black box, a proprietary secret that its developers are fiercely protective of. This lack of transparency has fueled considerable skepticism within the financial community.
The Skeptics' Corner: Doubts and Disagreements
Many experts remain unconvinced. The claims of unparalleled accuracy seem almost too good to be true. The model’s complexity is a major hurdle; even seasoned quantitative analysts are struggling to decipher its inner workings.
Overfitting: A Statistical Nightmare
One major concern is overfitting. Overfitting occurs when a model fits the historical data too perfectly, leading to poor predictions on new, unseen data. It's like memorizing the answers to a test instead of understanding the material; you ace the test but fail the exam. There's considerable debate whether the Solebury Model has fallen victim to this statistical trap.
Data Bias: A Crooked Mirror
Another criticism centers on potential biases in the data itself. The model uses a vast array of data sources, and biases within those sources could easily skew its predictions. Think of it as relying on a slightly warped mirror to see the future – the reflection might be close, but it's also subtly distorted.
The Investigation: Sifting Through the Evidence
Several independent investigations are underway to assess the Solebury Model’s true predictive power. Early results are…mixed, to put it mildly. Some analyses show impressive performance in backtesting (testing the model on historical data), while others reveal significant limitations when applied to real-time market forecasting.
The Backtesting Debate: Past Performance is Not Indicative of Future Results
The backtesting results are particularly contentious. Critics argue that impressive backtesting results can be misleading, especially with complex models that have many adjustable parameters. It's easy to create a model that fits past data perfectly, but that doesn't mean it will perform well in the future. Think of it as fitting a curve to a scatter plot; you can always find a curve that fits, but it might not be representative of the underlying relationship.
Real-World Application: The Ultimate Test
Ultimately, the true test of the Solebury Model will be its performance in live trading environments. Initial results from real-world applications are showing lower than expected accuracy, further fueling concerns about overfitting and data bias.
The Verdict? Still Out.
The investigation into the Solebury Model is far from over. The jury is still out on whether this innovative approach truly delivers on its promises or if it’s ultimately a statistical mirage. Transparency is key here; until the model’s inner workings are more openly scrutinized, doubts will persist. Perhaps the real story isn't about the model's accuracy but about the challenges of interpreting vast, complex datasets and the inherent limitations of predictive modeling in the chaotic world of finance.
This is not just about a model; it's about the ongoing debate between hype and reality in the world of financial technology. It’s a story that's still unfolding, and one worth following closely.
Frequently Asked Questions
1. Is the Solebury Model's source code publicly available? No, the source code is proprietary and confidential, adding to the skepticism surrounding its methodology and claims. This lack of transparency makes independent verification and validation extremely challenging.
2. What are the biggest risks associated with the Solebury Model? The biggest risks stem from potential overfitting, data bias, and the inherent limitations of attempting to predict the inherently unpredictable nature of financial markets. Over-reliance on this model could lead to significant financial losses.
3. How does the Solebury Model compare to other predictive models in finance? Direct comparisons are difficult due to the lack of transparency surrounding the Solebury Model. However, preliminary analysis suggests that its performance, at least in the current iteration, does not significantly outperform established models.
4. What regulatory scrutiny is the Solebury Model facing? Given the model's potential impact on financial markets, regulatory bodies are likely to scrutinize its methodology, claims, and potential risks. This could lead to future restrictions on its use or stricter regulations around the use of similarly complex AI models in finance.
5. What are the ethical implications of using such a complex, opaque model for financial predictions? The ethical implications involve issues around transparency, accountability, and the potential for misuse. The lack of transparency could lead to unfair advantages for certain parties and increase the risks for others. Moreover, the possibility of bias in the data used could lead to discriminatory outcomes.
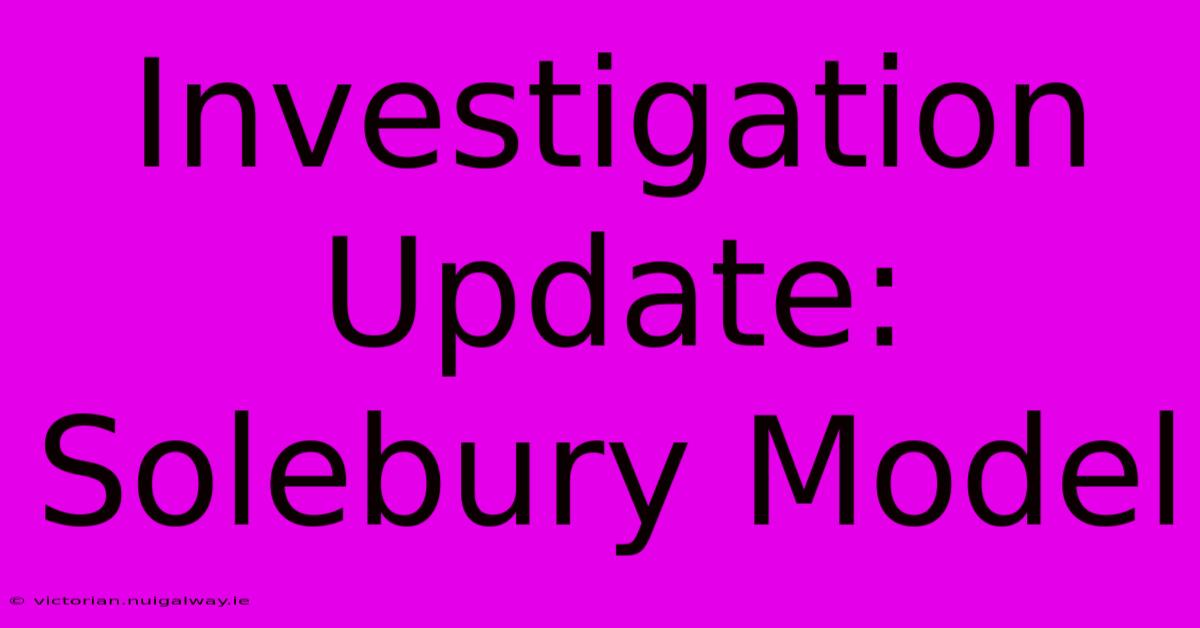
Thank you for visiting our website wich cover about Investigation Update: Solebury Model. We hope the information provided has been useful to you. Feel free to contact us if you have any questions or need further assistance. See you next time and dont miss to bookmark.
Also read the following articles
Article Title | Date |
---|---|
Tcu Vs Louisiana Tv Channels And Free Streaming Links | Dec 29, 2024 |
Tysons Record Broken After 38 Years | Dec 29, 2024 |
Miamis Ward Breaks Passing Td Record | Dec 29, 2024 |
Bahubali Nkr Mcg Box Office Report | Dec 29, 2024 |
Boxer Dies Days Post Fight Win | Dec 29, 2024 |
Bengals Week 17 Higgins Availability | Dec 29, 2024 |
Denchs Tree A Fruitful Tribute To Smith | Dec 29, 2024 |
Dayle Haddon Death Details Emerge | Dec 29, 2024 |
Littlers Tax Trick Big Savings | Dec 29, 2024 |
Nitish Reddy Andhras Emerging Leader | Dec 29, 2024 |