New Approach To Distortion Detection
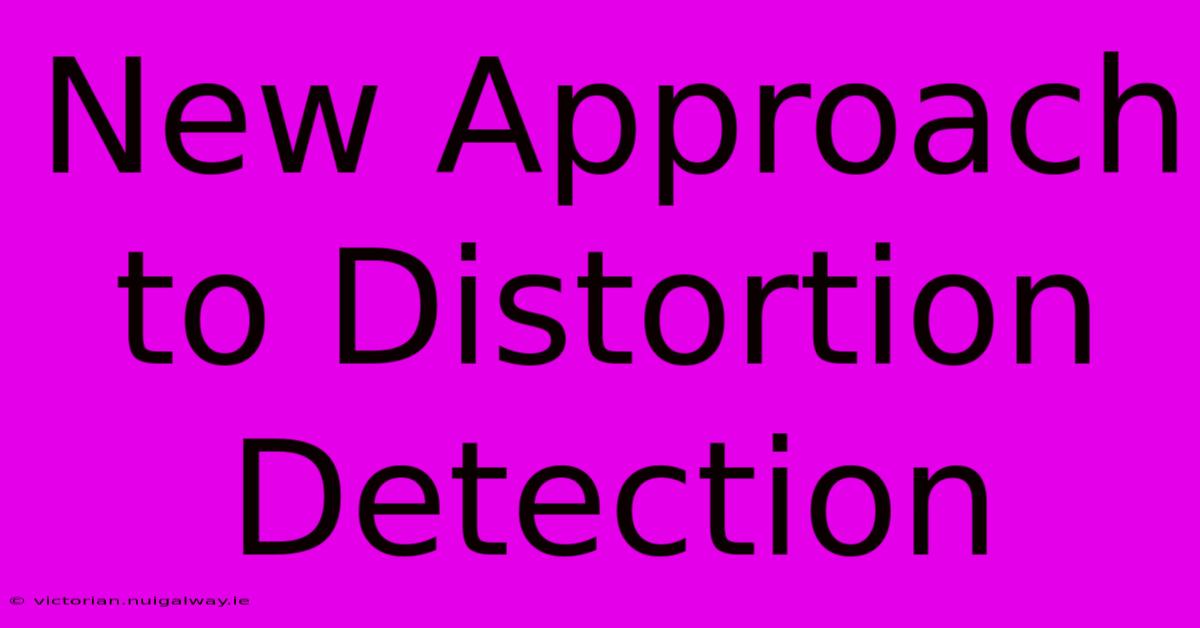
Discover more detailed and exciting information on our website. Click the link below to start your adventure: Visit Best Website. Don't miss out!
Table of Contents
A New Approach to Distortion Detection: Beyond the Pixel
Let's be honest, distortion detection is usually about as exciting as watching paint dry. We're talking about algorithms meticulously scrutinizing images, hunting for imperfections like a digital bloodhound. But what if I told you there's a whole new world beyond pixel-peering? A world where we can detect distortion not just by what we see, but how we see it? This is where our story begins.
The Tired Old Ways: Pixel-by-Pixel Purgatory
Traditional distortion detection methods are, to put it mildly, laborious. Think of those old-school image processing techniques – meticulously analyzing each pixel for inconsistencies, comparing it to its neighbors, and flagging any deviations. It's like finding a single misplaced Lego brick in a castle made of millions. Exhaustive, time-consuming, and frankly, boring. This approach, while effective in some cases, often struggles with subtle distortions or those cleverly masked by clever compression algorithms. It's like trying to catch a chameleon in a kaleidoscope – good luck!
The Limitations of Traditional Algorithms
These methods are frequently hampered by their reliance on predefined thresholds. What constitutes "too much distortion" is often subjective and depends heavily on the specific application. A slight lens distortion might be acceptable in a casual snapshot, but completely unacceptable in a high-precision satellite image. This rigidity makes them inflexible and prone to errors. Think of it like using a cookie-cutter to judge the quality of a sculpture – you're missing the whole artistic point!
Computational Cost: The Elephant in the Room
Another major drawback is the sheer computational cost. Analyzing every single pixel in a high-resolution image is computationally expensive, requiring significant processing power and time. This makes real-time distortion detection a challenge, particularly for applications that demand speed, such as live video streaming or autonomous driving systems. It's like trying to solve a million Sudoku puzzles simultaneously – your computer will probably melt!
A Paradigm Shift: Embracing the Human Element
So, how do we escape this pixel-by-pixel purgatory? The answer, my friends, lies in mimicking the human visual system. We don't analyze every single detail of an image; instead, we focus on the overall impression, the gestalt. We perceive distortions intuitively, noticing inconsistencies in shapes, perspectives, and textures. This is where the magic happens.
Bio-Inspired Algorithms: Learning from Nature
By developing algorithms inspired by the human visual cortex, we can create systems that are not only more efficient but also more robust. These algorithms can focus on identifying patterns and anomalies in the image's overall structure, rather than getting bogged down in individual pixel analysis. This approach leverages the power of machine learning and deep neural networks to learn what constitutes "normal" and flag deviations from that norm. It's like having an expert art critic analyze a painting – they'll notice subtle flaws that would escape a simple pixel-by-pixel scan.
The Power of Deep Learning: Detecting Subtle Distortions
Deep learning models, trained on vast datasets of distorted and undistorted images, can learn to identify subtle distortions that would go unnoticed by traditional methods. They can detect anomalies in textures, inconsistencies in perspective, and even slight shifts in color balance – all without the need for laborious pixel-by-pixel analysis. It's like having a superhuman with impeccable eyesight analyzing your images.
Beyond Images: Expanding the Horizons of Distortion Detection
The potential of this new approach extends far beyond simple image analysis. Imagine applying similar principles to other forms of data: audio signals, sensor readings, even financial time series. A subtle anomaly in a heartbeat monitor, a slight distortion in a network signal, or an unusual fluctuation in stock prices – all could be detected using these bio-inspired algorithms.
Applications in Diverse Fields: From Medicine to Finance
The applications are vast and varied. In medicine, this could revolutionize disease detection by identifying subtle abnormalities in medical images. In finance, it could help detect fraudulent transactions by identifying unusual patterns in financial data. And in engineering, it could improve the safety and reliability of critical systems by detecting anomalies in sensor readings.
The Future of Distortion Detection: A Collaborative Effort
Developing these advanced algorithms requires a collaborative effort between computer scientists, neuroscientists, and experts in various application domains. By combining the power of artificial intelligence with a deep understanding of human perception, we can create systems that are not only more accurate and efficient but also more adaptable and robust. This is not just about detecting distortions; it's about understanding them, learning from them, and ultimately, improving our world.
Conclusion: A New Era of Precision
The future of distortion detection lies not in pixel-by-pixel scrutiny, but in mimicking the power and elegance of the human visual system. By embracing bio-inspired algorithms and the power of deep learning, we can create systems that are faster, more accurate, and capable of detecting subtle distortions that were previously impossible to identify. This is more than just technological advancement; it's a paradigm shift that will reshape how we approach many fields, from image processing to medical diagnostics and financial analysis. The journey has just begun.
FAQs: Unraveling the Mysteries of Distortion Detection
1. Can these bio-inspired algorithms handle different types of distortions simultaneously? Yes, the beauty of these algorithms lies in their adaptability. They can be trained to recognize various distortion types simultaneously, making them far more versatile than traditional methods.
2. How do these algorithms handle noisy data? Advanced algorithms incorporate noise reduction techniques and utilize robust statistical methods to minimize the impact of noise on distortion detection accuracy. The training process itself often involves exposure to noisy data, making the algorithms more resilient.
3. What about the ethical implications of advanced distortion detection? This is crucial. Just as powerful technology like facial recognition needs ethical safeguards, so too do these algorithms. Bias in training data can lead to skewed results, highlighting the need for carefully curated and representative datasets.
4. What role does computational power play in this new approach? While deep learning models demand computational resources, advancements in hardware and optimization techniques are making these approaches increasingly feasible for real-time applications. We're constantly finding ways to make them more efficient.
5. How far off are we from seeing widespread adoption of these new techniques? We're already seeing implementations in specialized areas. Widespread adoption depends on further research, refinement of algorithms, and the development of user-friendly interfaces that can make this powerful technology accessible to a broader range of users.
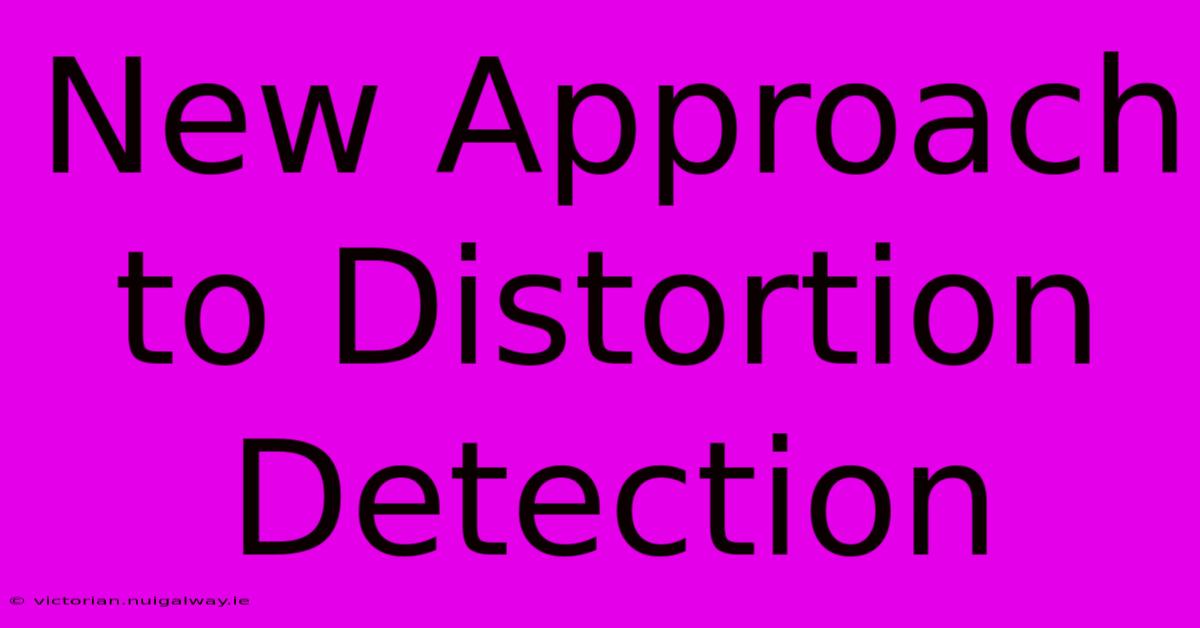
Thank you for visiting our website wich cover about New Approach To Distortion Detection. We hope the information provided has been useful to you. Feel free to contact us if you have any questions or need further assistance. See you next time and dont miss to bookmark.
Also read the following articles
Article Title | Date |
---|---|
James Woods Witnesses House Fire | Jan 09, 2025 |
Trump Plans Gulf Of Mexico Rename | Jan 09, 2025 |
Green Brigades Dundee Utd Demonstration Against Celtic | Jan 09, 2025 |
Illegal Release Highlands Lynx Captured | Jan 09, 2025 |
Carlton Presidents Social Media Accounts Compromised | Jan 09, 2025 |
Bradley Spurs Defeat Second Leg | Jan 09, 2025 |
La Wildfire Pacific Palisades Devastation | Jan 09, 2025 |
Actor James Woods Wildfire Ordeal | Jan 09, 2025 |
James Woods Palisades Fire Escape Story | Jan 09, 2025 |
Steve Guttenberg Helps La Fire Victims | Jan 09, 2025 |