Open Source LLM DeepSeek R1 Vs OpenAI O1
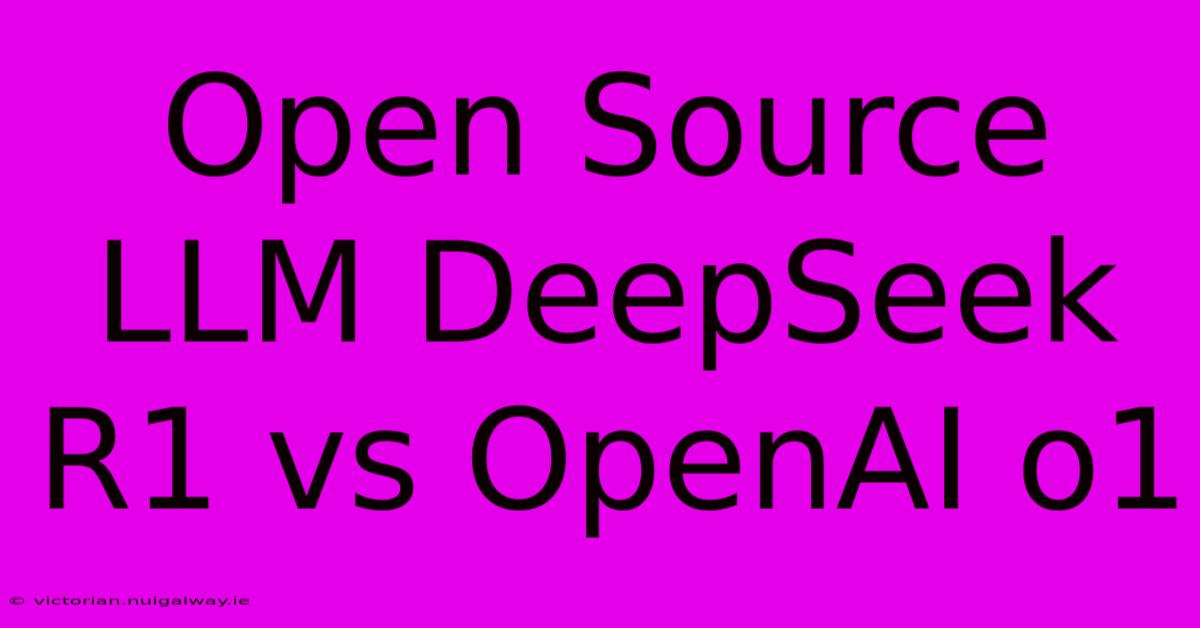
Discover more detailed and exciting information on our website. Click the link below to start your adventure: Visit Best Website. Don't miss out!
Table of Contents
Open Source LLM DeepSeek R1 vs OpenAI o1: A David vs. Goliath Showdown?
Hey there, fellow AI enthusiasts! Let's dive into a fascinating face-off: DeepSeek R1, a relative newcomer in the open-source Large Language Model (LLM) arena, versus the heavyweight champion, OpenAI's o1 (let's assume 'o1' refers to a hypothetical, future, advanced OpenAI model for the sake of this comparison โ adjust if a specific model exists). This isn't just a tech comparison; it's a story about innovation, accessibility, and the potential disruption of a giant.
The Challenger: DeepSeek R1 โ The Open Source Maverick
DeepSeek R1, with its open-source nature, immediately grabs our attention. Think of it as the scrappy underdog, ready to challenge the established order. Its strength lies in its community-driven development. Imagine a global team of developers, constantly tweaking, improving, and adding features. This collaborative spirit fosters rapid innovation and allows for a level of customization rarely seen in proprietary models.
DeepSeek R1's Unique Strengths: A Community-Powered Revolution
- Transparency and Auditability: Unlike closed-source models, DeepSeek R1's code is open for all to see. This allows for independent verification, reducing the "black box" problem and fostering trust.
- Customization and Adaptability: Need a model fine-tuned for a specific niche? With DeepSeek R1, you're empowered to do it yourself. This flexibility is a game-changer for researchers and businesses.
- Cost-Effectiveness: Open-source models often translate to significant cost savings, especially for smaller organizations or individual researchers who might not have the budget for expensive API calls.
DeepSeek R1's Potential Pitfalls: The Open Source Hurdles
- Maturity and Stability: Open-source projects, while innovative, sometimes lack the polish and stability of established commercial products. Bugs and inconsistencies are more likely.
- Maintenance and Support: Relying on a community for support can be unpredictable. While the collective knowledge is vast, getting timely assistance might be challenging.
- Security Concerns: While the open nature allows for scrutiny, it also exposes the model to potential vulnerabilities that malicious actors might exploit.
The Champion: OpenAI o1 โ The Proprietary Powerhouse
OpenAI's hypothetical o1 (or any comparable advanced model) represents the established champion. It's likely to boast superior performance on various benchmarks, refined through vast resources and rigorous testing. Think of it as the finely-tuned race car, built with cutting-edge technology and a massive budget.
OpenAI o1's Undisputed Advantages: The Goliath's Arsenal
- Performance and Accuracy: Expect higher accuracy rates, better fluency, and a deeper understanding of nuances in language. This is a direct result of massive datasets and sophisticated training techniques.
- Scalability and Reliability: OpenAI's infrastructure ensures stability and high availability, crucial for mission-critical applications.
- Robust Support and Documentation: Commercial models often come with comprehensive documentation and dedicated support teams, ensuring a smoother user experience.
OpenAI o1's Achilles Heel: The Proprietary Price Tag
- Cost: Access to powerful models like o1 often comes at a steep price, limiting access for many researchers and smaller companies.
- Lack of Transparency: The proprietary nature restricts access to the model's inner workings, creating a "black box" that limits customization and understanding.
- Vendor Lock-in: Reliance on a single provider can lead to vendor lock-in, making it challenging to switch platforms if needs change.
The Verdict: It's Not Just About the Numbers
Choosing between DeepSeek R1 and OpenAI o1 isn't a simple matter of comparing benchmark scores. It's a strategic decision based on your priorities. DeepSeek R1 offers freedom, customization, and cost-effectiveness, making it ideal for researchers, startups, and anyone who values transparency and community involvement. OpenAI o1 (or its equivalent) provides superior performance and reliability, but at a cost.
Think of it as choosing between a meticulously handcrafted, bespoke suit versus a perfectly fitting, mass-produced garment. Both serve the same purpose, but the experience, price point, and level of customization are vastly different.
The Future of LLMs: A Collaborative Landscape?
The ongoing competition between open-source and proprietary models isn't a zero-sum game. We might see a future where the strengths of both converge, fostering a more collaborative and innovative ecosystem. Imagine open-source projects building upon the advancements of proprietary models, or proprietary companies adopting open-source tools to enhance their own offerings. This synergistic approach could accelerate the progress of LLM technology, benefiting everyone.
FAQs: Unpacking the DeepSeek R1 vs. OpenAI o1 Debate
-
Beyond performance metrics, what are the ethical implications of choosing an open-source versus proprietary LLM? Open-source models promote transparency and accountability, potentially mitigating ethical concerns around bias and misuse. Proprietary models, while often subject to ethical guidelines, may lack the same level of public scrutiny.
-
How might the regulatory landscape impact the future of both open-source and proprietary LLMs? Future regulations might focus on transparency, bias mitigation, and data privacy, impacting both open-source and proprietary models. Open-source models might face challenges in ensuring compliance across their distributed development community.
-
Can open-source LLMs like DeepSeek R1 truly compete with the massive resources of companies like OpenAI in terms of model size and training data? While surpassing the scale of OpenAI might be a challenge, the open-source community's collaborative efforts and innovative techniques could lead to surprising breakthroughs in model efficiency and performance.
-
What role will community involvement play in the long-term success of open-source LLMs like DeepSeek R1? Community engagement is paramount. Active contributions in code development, testing, documentation, and community support are essential to ensure the continued growth and improvement of open-source LLMs.
-
How can businesses leverage the strengths of both open-source and proprietary LLMs within their operations? Businesses might use open-source LLMs for specific tasks or niche applications where customization is crucial, while leveraging proprietary models for large-scale, mission-critical applications that require high performance and reliability. The optimal approach is often a hybrid strategy.
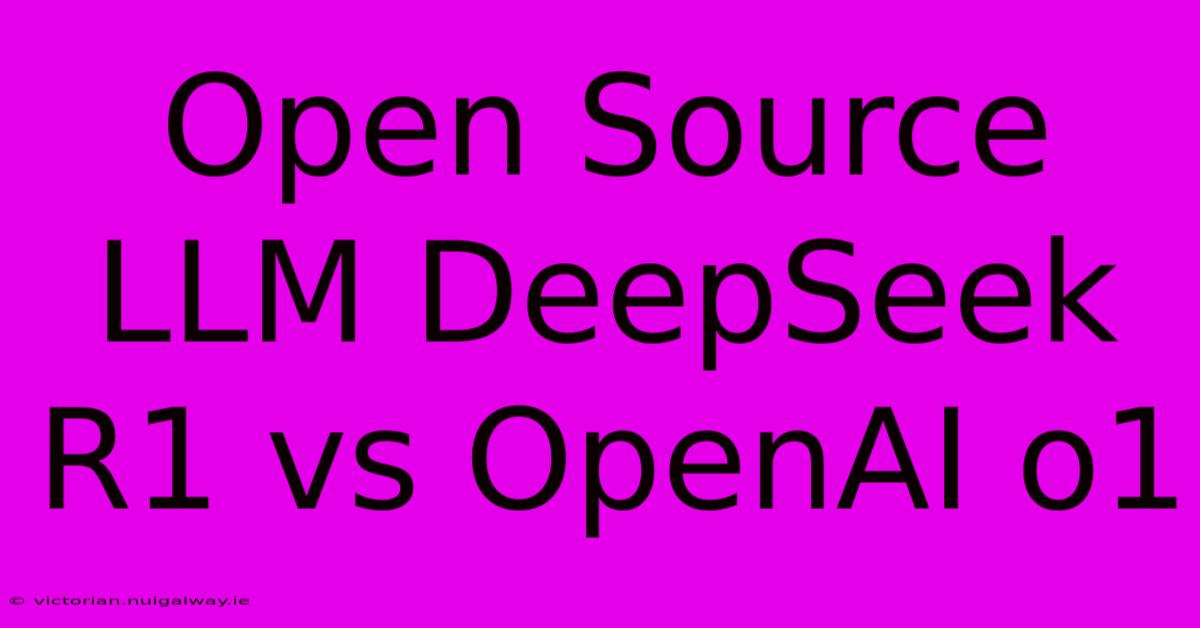
Thank you for visiting our website wich cover about Open Source LLM DeepSeek R1 Vs OpenAI O1. We hope the information provided has been useful to you. Feel free to contact us if you have any questions or need further assistance. See you next time and dont miss to bookmark.
Also read the following articles
Article Title | Date |
---|---|
Australia Day Celebrating Defence Personnel | Jan 26, 2025 |
Lean Year For Gulf Deepwater Oil Discoveries | Jan 26, 2025 |
La Fire Unexpected Trump Newsom Meeting | Jan 26, 2025 |
Deep Seek R1 Open Source Alternative To O1 | Jan 26, 2025 |
La Liga 2025 Valladolid Vs Real Madrid Lineups | Jan 26, 2025 |
Kelsey Parkers Pregnancy Announcement | Jan 26, 2025 |
Mbappe Red Real Madrids Valladolid Loss | Jan 26, 2025 |
Emergency Landing United Flight Injuries | Jan 26, 2025 |
Husbands Tactics Underdogs Ao Win | Jan 26, 2025 |
Sabalenka Falls To Keys In Grand Slam Final | Jan 26, 2025 |